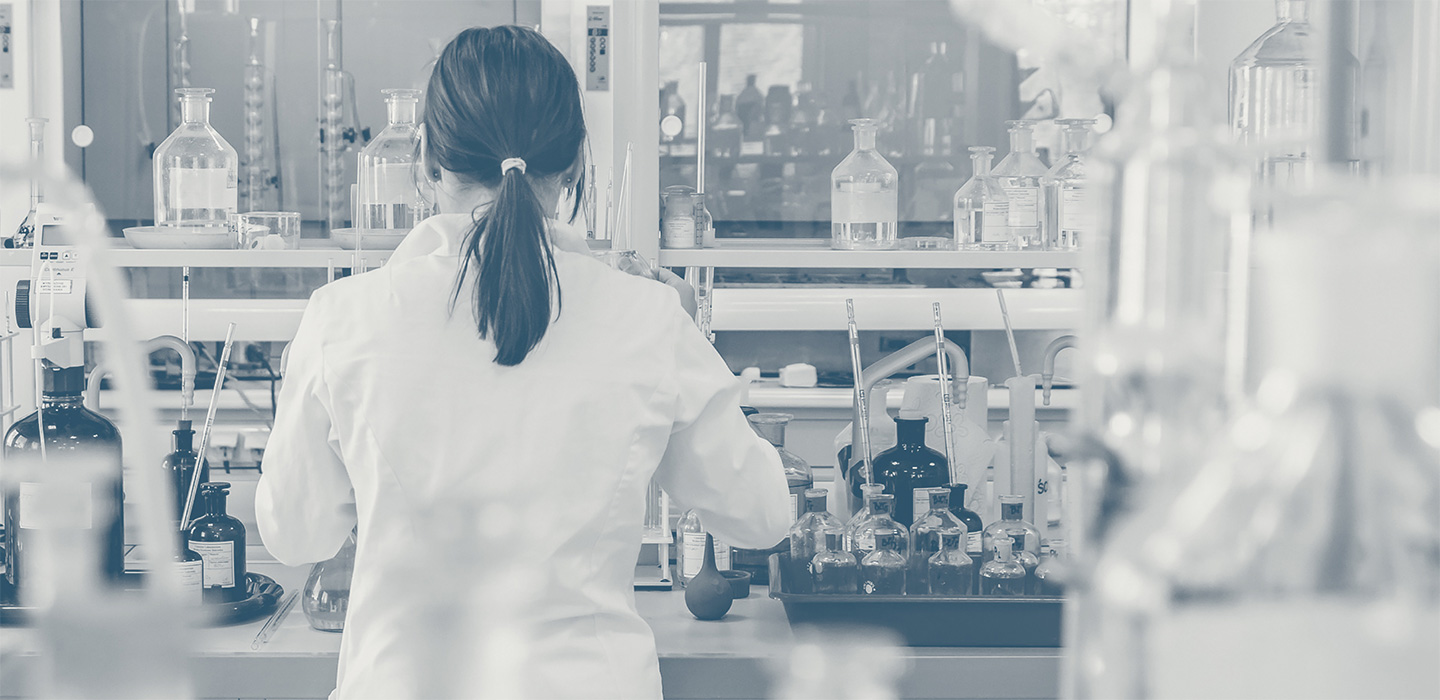
2021
Samantha J. Riesenfeld, PhD
Assistant Professor, Pritzker School of Molecular Engineering, University of Chicago
Extracting Latent Transcriptional and Multi-omic Features from Digital Pathology to Guide Cancer Treatment
What genomic and epigenetic information about cancer can data-driven artificial intelligence systems learn to extract from images of tumors?
Multi-omics data, which include genome-wide measurements of DNA, mRNA, chromatin accessibility, and proteins, offer potentially powerful ways to access the underlying complexity of cancer. Advances in genomic sequencing and computation have enabled personalized treatments that target single genetic alterations, yet such therapies often fail, suggesting that treatment responses are influenced by a larger multi-omics context. However, the expense and logistics of obtaining rich genomic information for each patient present major barriers to better understanding this context and using it to improve treatments. Instead, Dr. Riesenfeld proposes to infer underlying, hidden or “latent” transcriptional or multi-omic factors whose values in samples both distill the relevance of different molecular pathways or cell states and can be associated by artificial intelligence systems to tissue features in corresponding histological images.
Based on preliminary findings, Dr. Riesenfeld expects that “deep learning” networks (computational neural networks that make inferences from data without the aid of human supervision) may be trained to predict the values of some latent factors directly from digital pathology images of solid tumors. Once trained on large public datasets, these systems will be applied to test whether inferred latent factor values contain clinically relevant information, for example, explaining variation in treatment responses. The ability to extract complex, multi-omic information directly from imaging would both enable and equalize the detection and study of new molecular features of many cancers, allowing them to inform treatment decisions for a wide range of patients, potentially without the high cost of obtaining and analyzing genetic material from each patient. Dr. Riesenfeld’s work has the potential to provide a vast amount of information about cancer multi-omics and new insights into how such data can drive accessible, personalized treatments.